Traditional AI vs GenAI: Amplified Risks and Challenges in Governance Explained
- palmerlisac
- Nov 10, 2023
- 2 min read
I always want to help to demystify the AI journey, this article by Martijn Wiertz includes a deep dive explanation of how foundation models are different from conventional AI models and how that affects AI governance. Below, I pulled out the key takeaways including:
MLOps vs FMOps
Traditional AI vs GenAI amplified risks
Questions to guide foundation model selection
FM use case end-to-end governed workflow
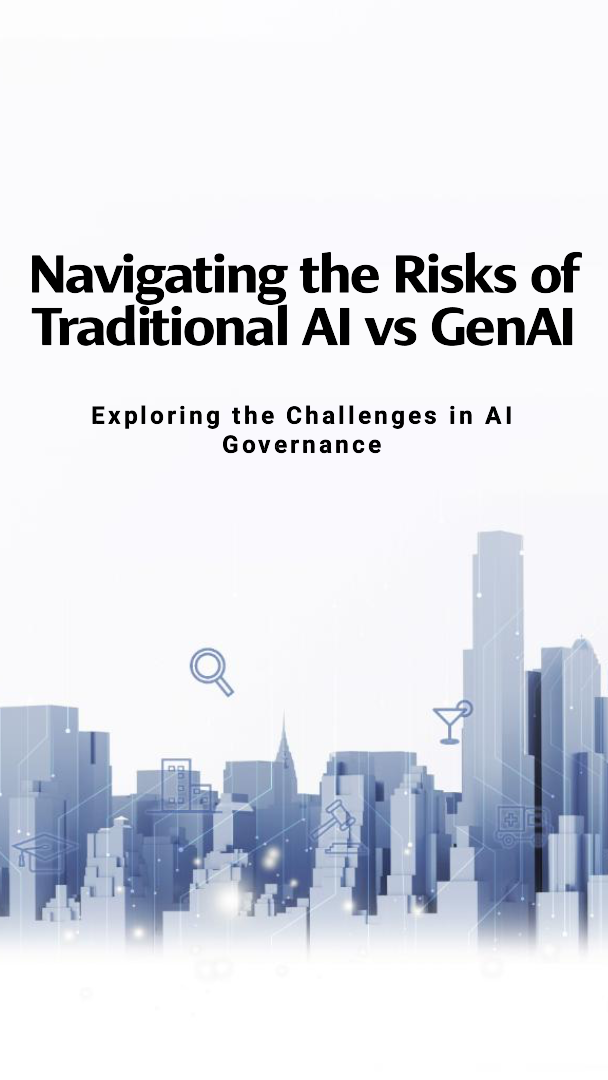
MLOps differs from FMOps
Foundation models are trained for multiple tasks, while conventional models are trained for a specific task.
Foundation models learn general representations, while conventional models learn task-specific patterns.
Foundation models are trained on large general datasets, while conventional models are trained on specific data.
Users can interact with foundation models through prompt engineering, prompt tuning, and fine-tuning.
Foundation models offer additional parameters like temperature, top-K, repetition penalty, and minimum token count.
Foundation models support tasks like summarization, entity extraction, and generative tasks.
Evaluation of foundation models requires different metrics like ROUGE and BLUE.
Traditional AI vs GenAI Amplified Risks
Risks associated with input:
Traditional risks: Legal restrictions on
Amplified risks: Copyright and other IP issues with the content.
New risks: Vulnerabilities to new types of adversarial attacks such as prompt injecting.
Risks associated with output:
Traditional risks: Performance disparity across individuals or groups.
Amplified risks: Challenges in explaining why output was generated.
New risks: Hallucination—false content generation.
Challenges:
Traditional risks: Documenting data and model details, purpose, potential uses and harms.
Amplified risks: Increased carbon emission due to high energy requirement to train and operate.
New risks: Homogenizing of culture and thoughts.
Foundation Model Selection Questions
When evaluating a foundation model candidate, ask these types of questions about that model:
What data sources are used to train the model?
How is the training data governed and processed?
How are socio-technical harms and risks mitigated?
What are the energy consumption and carbon emissions, for training and for inferencing?
What are the terms and conditions for using this model (e.g., will your data be used in (re)training of the model)?
What are the costs of using this model?
How is our data secured when we use this model?
What intellectual property indemnification does the model creator provide?
Which tasks does the model support, and at what quality?
Which of the supported tasks will we allow?
FM Use Case Governance Workflow
Model approval
Use case approval
Model selection
Model fine tuning
Prompt development
Evaluation and monitoring
Change requests
Conclusion
Understanding the differences between foundation models and conventional AI models is crucial for effective AI governance. The amplified risks associated with GenAI, such as copyright issues and vulnerabilities to new adversarial attacks, call for a more comprehensive risk management strategy.
Foundation model selection requires careful consideration of data sources, governance processes, socio-technical harms, energy consumption, and vendor terms and conditions (anyone who follows my writing knows that T&Cs is a topic near to my heart - be careful what you agree to). Finally, the FM use case workflow serves as a useful guide for implementing responsible AI practices.
By addressing the challenges and asking critical questions, organizations can navigate the AI journey with a focus on business value creation within a responsible deployment framework.
Please reach out to my team if you need help tackling this fast-evolving environment! Lisa@drlisa.ai
Comments