5 Strategic AI Governance Priorities Every CIO/CAIO Must Own
- palmerlisac
- 6 days ago
- 7 min read
This guide was originally developed in response to a request for insights from CIO.com. I've expanded and adapted here to share more deeply with my broader network.
By Dr. Lisa Palmer, Former CIO, CEO of Dr. Lisa AI and NCX (AI-Powered Human Expertise Startup) - April 13, 2025
“AI governance can’t be thought of as slowing innovation. AI Leaders should use governance to turn AI into real business value, while protecting the enterprise from legal and operational blind spots.”
Why AI Governance Is a C-Suite Imperative
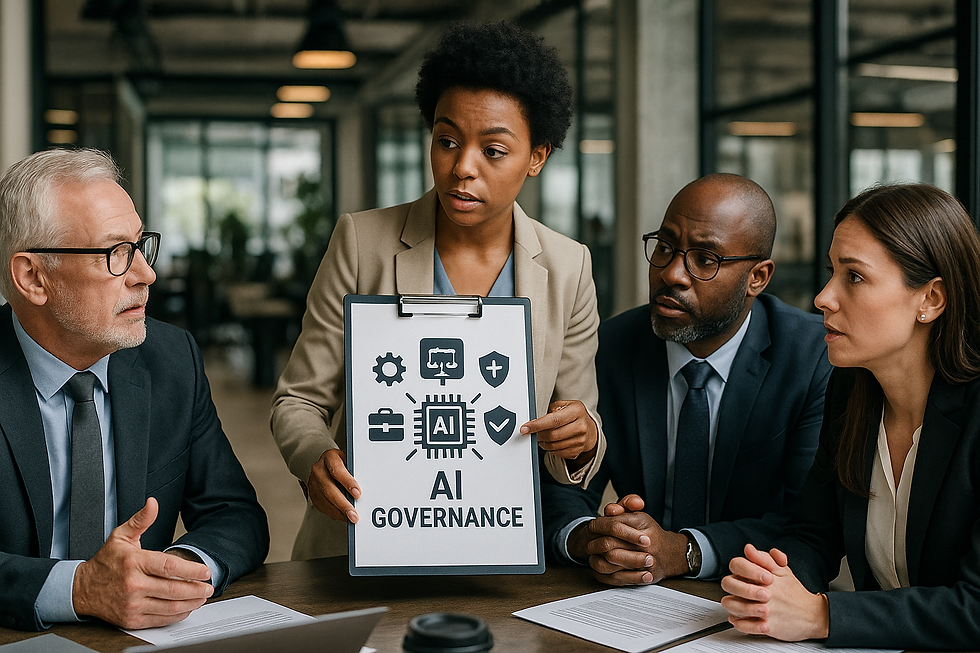
As artificial intelligence transitions from experimental pilots to enterprise-wide deployments, AI governance has become a strategic priority for Chief Information Officers (CIOs). It’s no longer just a compliance or technical checkpoint—it’s a core business capability that impacts innovation velocity, organizational trust, and board-level risk exposure.
In some organizations, this responsibility falls to a Chief AI Officer (CAIO) or another AI-aligned executive. Regardless of title, the imperative remains the same: someone at the executive level must own the strategy, structure, and accountability behind how AI is governed at scale.
Yet, while many talk about governance in theory, few connect it to measurable outcomes or actionable leadership. In this piece, I outline five governance priorities CIOs must lead—and show you how to turn governance into a driver of business value, not just an oversight function.
Note: The tools mentioned below reflect current market leaders, though the landscape is evolving rapidly. Organizations should evaluate solutions against specific use cases and avoid governance silos by ensuring tight integration across their tech stack.
1. Use Governance to Anchor AI in Business Value and Customer Impact
CIOs should be using governance to treat AI as a critical business asset. Effective AI governance ensures every initiative is aligned with real business problems, delivers measurable outcomes, and advances customer impact. Too often, governance is treated as a compliance function. Instead, it should be a strategic, action-oriented decision-making framework that helps CIOs filter out “toy AI” and focus resources on initiatives that drive growth and differentiation.
Think of it like managing a product portfolio: as CIO, you don’t greenlight every technology just because it’s new—you carefully select what enters the roadmap, based on strategic alignment and potential ROI. Governance enables that same rigor in AI. It’s how CIOs prioritize, monitor, and scale AI investments—actively managing AI as a business asset, not a passive tool.
AI Leaders must also measure the ROI of governance itself. Effective AI governance reduces downstream costs from model rework, mitigates regulatory fines, accelerates time-to-market by enabling faster approvals, and safeguards brand trust. Metrics like “time-to-compliance,” “governance efficiency,” or “cost of risk realization,” can help quantify the value of governance to the enterprise (details at the bottom of the document). For strategic IT leaders, governance is not just a risk buffer—it’s a business enabler that deserves visibility on the performance dashboard.
Tools to explore: The ideal solution often involves integrating different tools. For example, using Fiddler/TruEra for deep model insights, DataRobot/H2O.ai for the ML lifecycle, and potentially an SPM tool like OnePlan or features within OneTrust/Monitaur for the overarching strategic business alignment and portfolio view.
2. AI Transparency and Human Accountability
“If CIOs aren’t holding vendors and internal teams accountable for AI transparency and human accountability, they’re leaving a critical gap in governance.”
Let’s take an example from healthcare. The human impact stakes are extremely high in this industry. If a CIO is implementing an AI-powered diagnostic tool for radiology, she needs to ensure transparency and accountability, there are two key steps:
With vendors, CIOs should require explainability features—such as heatmaps or annotated imaging—that show why a diagnosis was made. They must also demand transparency into training data and model performance across different patient populations to detect any potential bias, and embed accountability into contracts through SLAs and shared responsibility for patient safety.
Internally, CIOs should assign both a clinical and data science lead to oversee implementation and monitoring. These roles ensure AI outputs are reviewed alongside human expertise, and that anomalies—like disproportionate flags for specific demographic groups—trigger immediate review. Scheduled audits should be built into governance from the start.
This kind of structured transparency and accountability ensures AI supports clinical decision-making. This is the human + AI partnership that I counsel clients about every day, ensuring that risks are actively managed rather than discovered too late.
Tools to explore: Tools like Fiddler AI and TruEra (providing deep explainability, bias detection, and performance monitoring) alongside platforms like DataRobot and H2O.ai (offering integrated explainability and MLOps features across the lifecycle) provide critical technical capabilities. They enable organizations to understand model behavior, monitor for fairness and performance issues, and establish the necessary foundations for effective human accountability and oversight in AI systems.
3. Legal and Regulatory Compliance
“AI governance can’t stop at internal controls— CIOs need to be ready for AI-enabled influence at scale—where customers (or employees) can coordinate mass complaints, trigger regulatory reviews, or catalyze lawsuits with just a few clicks.”
In addition to classic internal governance challenges, governance frameworks must now also account for reputational and legal exposure created by AI outside your walls.
CIOs should:
Augment governance tools with adversarial-use detection (e.g., input pattern analysis, NLP-based coordination signals).
Prioritize real-time analytics to preempt reputational and regulatory risk.
Document and track AI-driven complaint resolutions to demonstrate compliance and build institutional trust.
Form an AI Crisis Task Force with legal, PR, and compliance. Draft pre-approved rebuttals for AI-driven misinformation and trigger internal audits when complaint volumes exceed thresholds.
Tools to explore: Credo AI (establishing internal compliance foundations and auditability), Case IQ (managing and documenting the response to AI-related incidents at scale), and Nightfall AI (preventing data-related compliance breaches in AI interactions) provide key capabilities. They help organizations prepare for external scrutiny, manage responses effectively, and mitigate the unique legal and regulatory risks amplified by AI.
4. Continuous Learning and Strategic Adaptation
“AI governance can’t be addressed like a static checklist. It’s a living system. CIOs need feedback loops, not fixed frameworks, to keep pace with shifting risks, regulations, and business goals.”
AI governance can’t be static—what works today likely will be outdated tomorrow. CIOs must lead with a mindset of continuous learning, where governance frameworks evolve alongside models, regulations, and business needs.
This means building feedback loops into every AI initiative:
Monitor model performance and behavior in the wild
Audit for bias and unintended consequences
Adjust policies and retrain systems as the landscape shifts
Maintain a cross-functional, broad human perspective team actively engaged in evolving governance
Think of it as governance as an ongoing process—not a one-time checklist. Organizations that adapt quickly will outperform those that treat governance as a set-it-and-forget-it function.
The most resilient AI strategies are the ones designed with adaptive, iterative discipline.
Tools to explore: Arthur AI (for critical feedback loop), ModelOp (for automating governance and adaptation across the lifecycle), and Dataiku (for providing an integrated environment for development, deployment, and ongoing management) are the kinds of technologies needed to implement a dynamic, adaptive, and continuous approach to AI governance. They provide the infrastructure to make AI governance a "living system."
5. Board-Level Communication
“CIOs carry the weight of AI risk. Without strong governance, they’re not just exposing the enterprise—they’re putting their own credibility on the line.”
Governance requires funding, staffing, and long-term commitment. To secure support, CIOs must communicate governance outcomes in language the board understands—like risk avoidance (like regulatory violations or costly model failures) and value created ((like faster time-to-market or sustained stakeholder trust).
This means going beyond dashboards. CIOs should:
Report on both risks avoided and value created
Tie governance metrics to business KPIs
Frame governance as a driver of safe acceleration and brand protection
Making Governance Measurable: ROI Metrics That Matter
“Governance shouldn’t just check boxes—it should create value. CIOs must measure governance not as a cost of compliance, but as a strategic tool for accelerating trust, performance, and business impact.”
To turn governance from a burden into a business asset, CIOs must make it measurable. The following three metrics—Time-to-Compliance, Governance Efficiency, and Cost of Risk Realization (CoRR)—offer a practical framework for quantifying impact. Each metric varies in complexity and is suited to different stages of organizational maturity. Time-to-Compliance is ideal for teams starting to formalize governance processes. Governance Efficiency is well-suited for organizations looking to optimize workflows and justify investments. CoRR requires more advanced tracking and coordination but provides the most direct insight into the financial value of risk mitigation. Together, these metrics help CIOs demonstrate that governance isn’t overhead—it’s a strategic enabler of trust, velocity, and resilience.
1. Time-to-Compliance
For organizations beginning to formalize governance processes, this is one of the most accessible and impactful metrics. It helps quantify how governance affects deployment velocity and identifies friction early in the lifecycle.
Tracks: Time from model submission to governance-approved deployment
Maturity: Beginner to Intermediate
Difficulty: Low to Medium
Example: Before implementing clear development standards and automated policy checks, the average Time-to-Compliance for models was 8 weeks, with 30% requiring significant rework after review. After implementation, the average drops to 6 weeks, with only 15% needing major rework, showing that better upfront alignment speeds up the compliant path to deployment.
Value: Directly links governance effectiveness to the speed of deploying trusted AI. Shorter times mean faster realization of business value from compliant models. It shows if governance is successfully shifting from a bottleneck to guardrails integrated earlier in the lifecycle.
2. Governance Efficiency
As AI initiatives scale, efficiency becomes critical. This metric helps CIOs assess how much time and effort governance is consuming—and where to streamline with automation or process redesign.
Tracks: Time, cost, and effort required for governance tasks (e.g., audits, reviews)
Maturity: Intermediate
Difficulty: Medium
Example: The pre-deployment review for high-risk models involves Legal, Compliance, InfoSec, and AI Ethics reviewers. Tracking shows it takes an average of 5 weeks and consumes ~70 person-hours per model. After implementing a new governance platform with automated checks and clearer workflows, the average time drops to 3 weeks and ~50 person-hours. This demonstrates improved Governance Efficiency.
Value: Identifies bottlenecks, justifies investments in governance tooling/automation, and ensures the process itself supports reasonable innovation velocity.
3. Cost of Risk Realization (CoRR)
This is the most advanced, but also the most strategic metric. CoRR helps organizations tie governance directly to financial impact, making it a powerful tool for board-level conversations and long-term planning.
Tracks: Financial impact of governance failures (e.g., fines, outages, data breaches)
Maturity: Advanced
Difficulty: High
Example: A company deploys a customer service chatbot that inadvertently leaks sensitive customer data. The CoRR calculation might include: $200k in regulatory notification costs and potential fines + $50k in external cybersecurity investigation fees + $80k in engineering time to fix and re-secure + $30k in customer support overtime and appeasement offers = $360k CoRR for that incident.
Value: Shows the tangible financial damage prevented by effective governance. Tracking CoRR over time can demonstrate if governance improvements are reducing the frequency/severity of costly failures.
Final Thoughts
Whether it’s the CIO, CAIO, or another AI-aligned leader, what matters most is that governance has a clear executive owner—and a seat at the strategy table. AI governance isn’t just a technical discipline—it’s a strategic leadership function. CIOs who treat it that way will:
Accelerate trusted, scalable innovation
Build board-level confidence
Demonstrate measurable ROI
And most importantly, set the tone for how AI is used responsibly across the enterprise
If you’re building or refining your AI governance strategy—and want to explore how to embed measurable value, executive alignment, and board-level readiness—my team and I would be happy to help. Reach us at josh@drlisa.ai.
Comments