Business Leaders Need to be Asking for Agentic AI
- palmerlisac
- Aug 5, 2024
- 10 min read
Updated: Aug 6, 2024
Keeping up with AI advancements is crucial for driving your business and staying competitive. The next big wave that I'm excited about is the shift from traditional Retrieval-Augmented Generation (RAG) to the more advanced Agentic RAG. This change will make AI work smarter and more effectively across various industries, reshaping applied AI and driving business innovation. Below, I explore the current state, key differences, benefits, and specific industry applications with practical examples and recommended actions, including scenarios from oil and gas, tax and audit, sports entertainment, healthcare, legal, financial services, and the public sector. And rest assured, I keep the content focused on business impacts, not technical jargon.
Current State of AI: The Prevalence of RAG
Retrieval-Augmented Generation (RAG) is arguably the most popular approach in Applied AI today. It's being used in every industry and across the public sector. It works by taking a user request and processing it through several steps to find, sort, and combine relevant information. This method ensures that the answers provided are accurate and contextually relevant, making it a powerful tool for organizations seeking to leverage AI for better decision-making. Think of it as a smart assistant that not only finds information for you but also ensures that it's accurate and easy to understand.
AI Statistics
Here are some key statistics that highlight the significant impact of AI in business and why it’s so critical to engage with this technology.
AI Market Growth: The AI market is projected to reach $407 billion by 2027, up from $86.9 billion in 2022.
Economic Impact: AI is expected to contribute a 21% net increase to the United States GDP by 2030.
Business Adoption:
Oil and Gas:
The global AI in oil and gas market will reach $5.70 billion by 2029, growing at a CAGR of 12.61%.
92% of oil and gas enterprises worldwide have either started investing in AI or plan to do so within the next two years.
In Texas, the largest oil-producing state in the US, there's expected to be a 27% increase in AI and data science jobs over the next decade.
Tax and Audit:
8% of tax firms are currently using generative AI technology; 13% of firms are planning to use AI technology soon; and 30% of tax and accounting firms are in the consideration phase for using generative AI tools.
79% of tax professionals who are aware of AI in their workplace say it's beneficial to have AI assist them with their job.
Sports Entertainment:
The sports technology industry, which includes AI applications, is expected to be worth approximately $48.7 billion by 2028, growing at a rate of 16.8% each year.
90% of sports executives believe AI will fundamentally impact the sports industry.
AI in sports is projected to create $2.6 trillion in value across various applications.
Healthcare:
The AI in healthcare market is projected to grow from $20.65 billion in 2023 to $187.95 billion by 2030, with a 37% annual growth rate from 2021 to 2022.
60% of U.S. adults would feel uncomfortable if their healthcare provider relied on AI for diagnosis and treatment recommendations.
The share of physicians' time spent on treating patients increased from 50% before AI implementation to 67% after AI implementation.
Legal:
The AI in legal market is projected to grow to $14 billion by 2027.
51% of law firms report using AI within their organization in some capacity.
AI can reduce legal research time by up to 80%; AI can aid in predicting litigation strategies with up to 90% accuracy; and legal chatbots can handle 60% of client queries.
Financial Services:
In 2023, the financial services industry invested an estimated $35 billion in AI, with banking leading the charge, accounting for approximately $21 billion.
69% of respondents used AI for data analytics in 2023, making it the most common application.
United States Public Sector:
As of 2020, approximately 150 federal government programs were using AI to assist with decision making and predictions based on data and algorithms. This number is continuing to rise.
The U.S. ranks high in the Government AI Readiness Index worldwide.
AI is being used across various levels of government, including municipal, county, state, and federal. Key areas of application include law enforcement, public benefits distribution, fraud detection, permitting, employment, and housing.
These statistics underscore the significant growth, economic impact, and business benefits of AI, making a compelling case for the transition to more advanced AI technologies like Agentic RAG.
Transition to Agentic RAG: What’s Different?
Agentic RAG takes AI to the next level. Unlike traditional RAG, which follows a simple, linear process, Agentic RAG uses a team of specialized agents to handle more complex tasks. Here's how it works:
Document Agents: Each document has its own dedicated agent that answers questions and summarizes information within its scope.
Meta-Agent: A top-level agent manages all the document agents, combining their outputs to create a coherent and comprehensive response.
This shift to Agentic RAG makes information processing and decision-making much more flexible and dynamic.
How Agentic RAG Enables Next Level AI Capabilities
Now that we understand what's different moving from RAG to Agentic RAG, let's explore how Agentic RAG takes AI improves capabilities by using independent and specialized agents to handle complex tasks. Here's how its unique features of independence, flexibility, initiative, self-improvement, and scalability, among others, make it a powerful tool for businesses.
Independence - Agentic RAG systems are built with independent agents that can retrieve, process, and generate information on their own. Think of it like having a team of experts who can work independently without needing constant supervision. This independence boosts efficiency and cuts down on the need for manual intervention.
Flexibility - Agentic RAG is highly flexible, allowing the system to adjust its strategies based on new data and changing contexts. Imagine a GPS that not only recalculates routes when you take a wrong turn but also adjusts to traffic conditions and road closures in real-time. This keeps the AI relevant and accurate over time.
Initiative - Agents in the Agentic RAG framework can anticipate needs and take preemptive actions to meet goals. Picture a personal assistant who not only schedules your meetings but also reminds you of important deadlines and preps necessary documents ahead of time. This forward-thinking approach is a major step up from traditional, reactive systems.
Self-Improvement - Agentic AI leverages advanced AI and machine learning to continually improve its understanding and responses. Picture a chess player who studies every game they play, learning new strategies and tactics to become a more formidable opponent over time. Using techniques like deep learning, reinforcement learning, and meta-learning to learn from interactions and become smarter over time, these systems become increasingly intelligent and effective.
Scalability - Agentic RAG systems are designed to scale seamlessly, managing increased workloads and expanding their capabilities without degradation in performance. Imagine a system that can handle an influx of users and new data without missing a beat, ensuring continuous efficiency and reliability.
With these advanced features and others, Agentic RAG offers significant improvements in efficiency, accuracy, and proactive decision-making. Next, let's explore how these capabilities translate into real-world applications across various industries.
The Impact of Agentic RAG in Various Industries
Agentic RAG is evolving how different industries leverage AI for better decision-making, efficiency, and new customer experiences. Below, I dig into how this approach creates valuable business outcomes across sector examples: oil & gas, tax & audit, the sports fan experience, healthcare, legal, financial services, and emergency response in public sector. I cover suggested use cases, examples of what the agents do, business outcomes that are created, and possible actions to get started.
1. Oil and Gas Industry: Reservoir characterization and production optimization
Implementation Setup: Integrate Agentic RAG with geological and production data systems.
Document Agents:
Geological Report Agent: Analyzes geological surveys and formation data.
Seismic Data Agent: Interprets seismic imaging results.
Well Log Agent: Analyzes data from existing wells in the area.
Production History Agent: Tracks and interprets historical production data.
Research Agent: Summarizes academic and industry research on similar formations.
Meta-Agent:
Synthesizes data from all document agents.
Generates comprehensive reservoir models.
Identifies optimal drilling locations and production strategies.
Produces a detailed report with production forecasts and optimization recommendations.
Outcome: Engineers receive comprehensive reservoir models, production forecasts, and optimization strategies. This leads to more accurate reserve estimates, optimized drilling locations, and improved production rates, ultimately increasing operational efficiency and profitability.
Getting Started: Implement Agentic RAG for a specific field or formation to test its impact on reservoir modeling and production optimization.
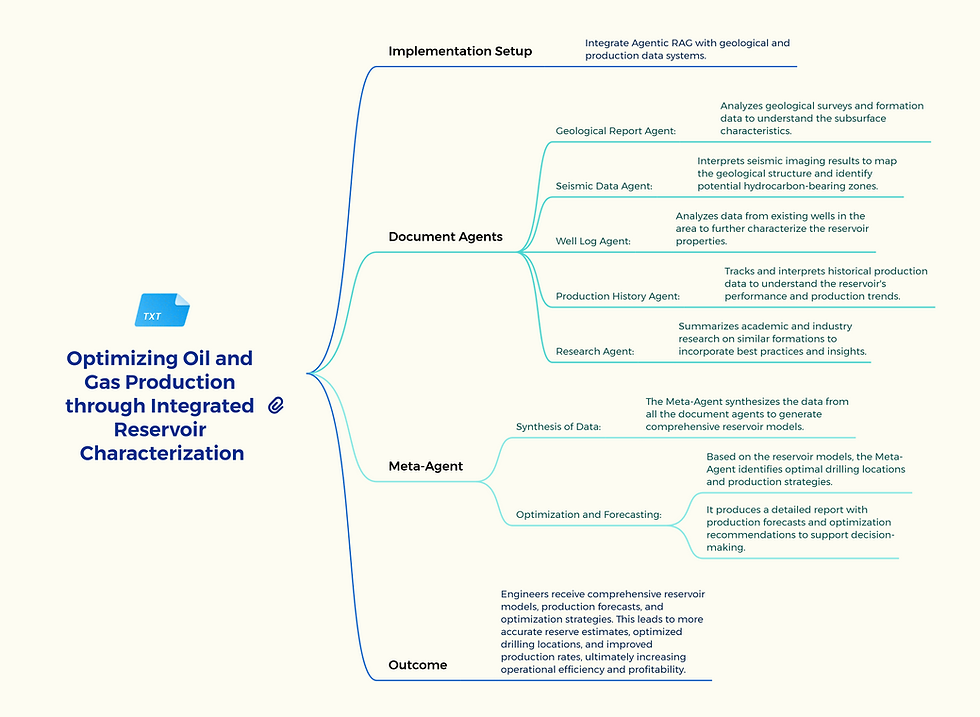
2. Tax and Audit Firms: Complex tax strategy development and risk assessment
Implementation Setup: Connect Agentic RAG with the firm's tax databases and client financial systems.
Document Agents:
Tax Code Agent: Analyzes relevant tax laws and regulations across jurisdictions.
Client Financial Agent: Interprets client's financial data and business operations.
Case Law Agent: Searches for and summarizes relevant tax court decisions.
Regulatory Guidance Agent: Tracks and interprets official guidance from tax authorities.
Meta-Agent:
Integrates information from all document agents.
Identifies tax optimization opportunities and potential risk areas.
Develops comprehensive tax strategies tailored to the client's situation.
Generates a detailed report with tax planning recommendations and compliance guidelines.
Outcome: Tax professionals receive detailed tax planning strategies, potential risk areas, and compliance recommendations. This results in more effective tax optimization for clients, reduced audit risks, and improved compliance with complex, ever-changing tax regulations.
Getting Started: Apply Agentic RAG to a high-value client's tax strategy to measure improvements in compliance and optimization.
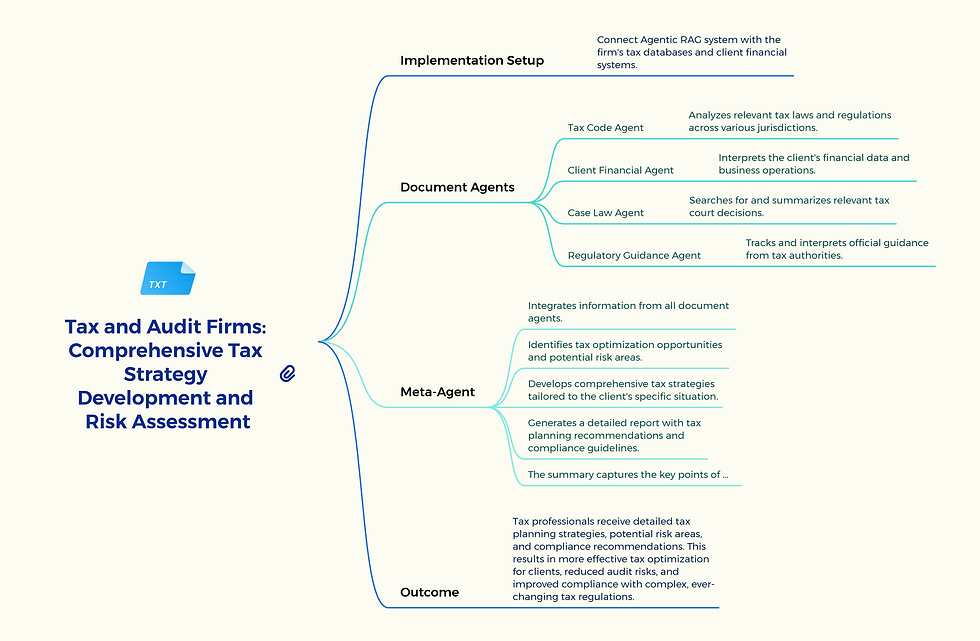
3. Sports Entertainment Fan Experience: Real-time, personalized sports content and analysis
Implementation Setup: Integrate Agentic RAG with live sports data feeds and fan interaction platforms.
Document Agents:
Live Game Data Agent: Analyzes real-time game statistics and events.
Historical Stats Agent: Provides relevant historical data and records.
Player Profile Agent: Gathers and interprets player performance and background information.
Social Media Agent: Tracks and analyzes relevant social media trends and fan reactions.
User Preference Agent: Analyzes individual fan's interaction history and preferences.
Meta-Agent:
Synthesizes information from all document agents.
Generates personalized insights and predictions based on user preferences and game context.
Creates interactive content tailored to the fan's interests.
Produces real-time, personalized commentary and analysis for enhanced fan engagement.
Outcome: Fans receive personalized real-time insights, predictive analysis, and interactive content tailored to their preferences. This leads to increased fan engagement, improved viewer retention for broadcasters, and new monetization opportunities for sports organizations through targeted offerings.
Getting Started: Launch a pilot program for a major sports event for box seat fans, using Agentic RAG to provide personalized content and measure its impact on fan engagement.
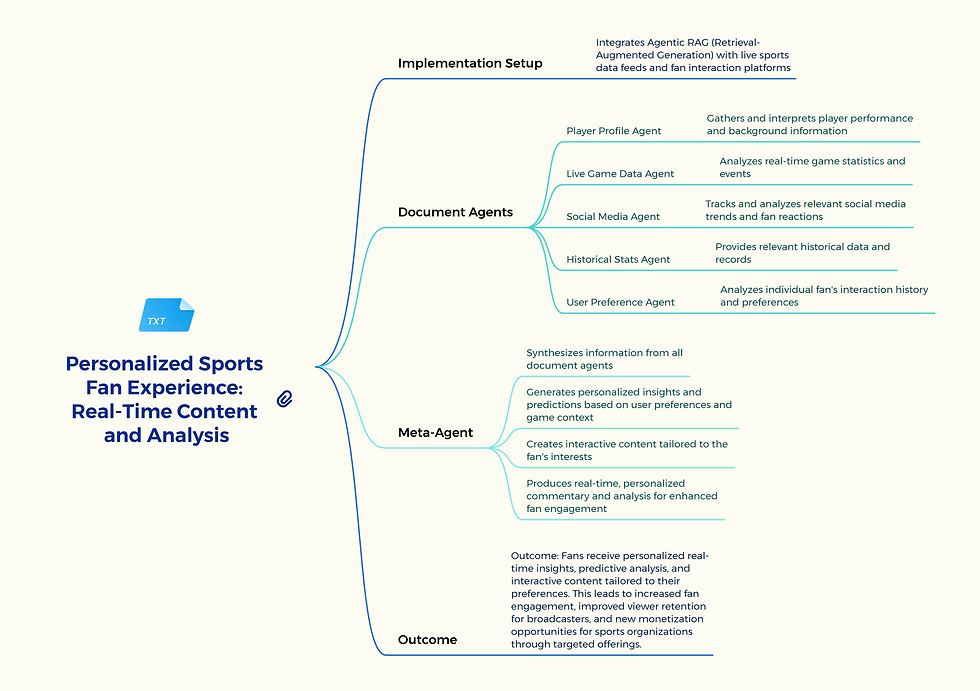
4. Healthcare Industry: Comprehensive patient diagnosis and treatment planning
Implementation Setup: Integrate Agentic RAG into the hospital's existing electronic health record (EHR) system.
Document Agents:
Patient History Agent: Analyzes patient's medical records, previous diagnoses, and treatments.
Lab Results Agent: Interprets recent and historical lab test results.
Medical Literature Agent: Searches and summarizes relevant medical research and clinical guidelines.
Treatment Protocol Agent: Identifies standard and experimental treatment options for similar cases.
Meta-Agent:
Integrates information from all document agents.
Identifies correlations between patient symptoms, lab results, and potential diagnoses.
Generates a comprehensive report with suggested diagnoses and personalized treatment plans.
Highlights areas requiring further investigation or specialist consultation.
Outcome: Doctors receive a detailed analysis that compares the patient's condition with similar cases, suggests potential diagnoses, and recommends personalized treatment plans. This leads to more accurate diagnoses, better-informed treatment decisions, and improved patient outcomes.
Getting Started: Implement a pilot program in a hospital department to integrate Agentic RAG into your diagnostic process, starting with a specific type of condition (e.g., cardiac diseases).
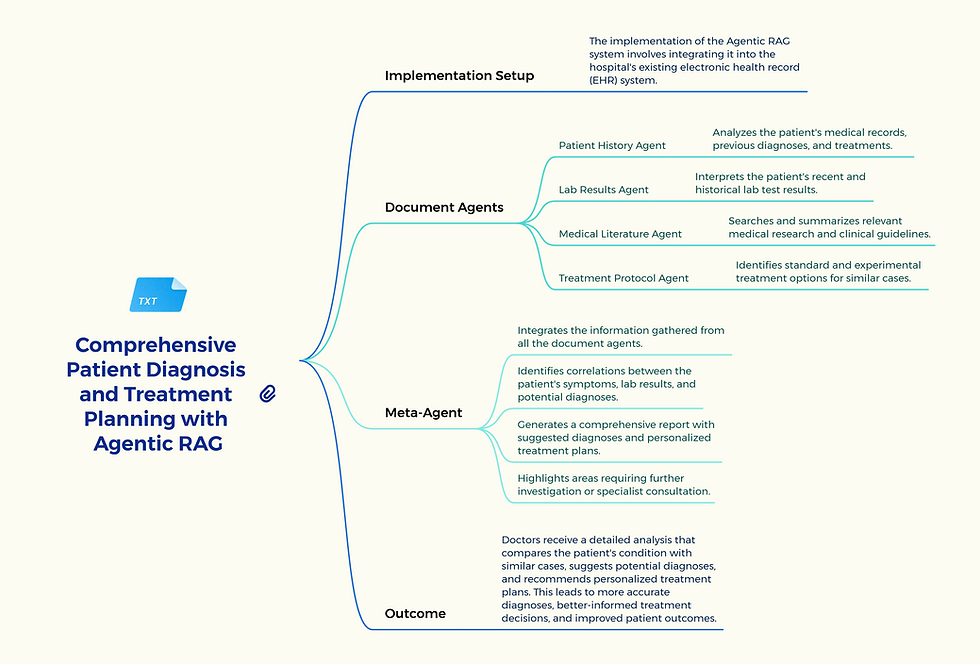
5. Legal Industry: Case law analysis and legal strategy development
Implementation Setup: Connect Agentic RAG with the firm's legal research database and case management system.
Document Agents:
Case File Agent: Analyzes the current case details and client information.
Precedent Agent: Searches for and summarizes relevant legal precedents.
Statute Agent: Identifies and interprets applicable laws and regulations.
Legal Commentary Agent: Gathers expert opinions and academic analyses on similar cases.
Meta-Agent:
Synthesizes information from all document agents.
Identifies strengths and weaknesses in the case.
Develops potential legal arguments and counterarguments.
Generates a comprehensive case strategy report with recommended actions.
Outcome: Lawyers receive in-depth analysis of relevant precedents, potential arguments, and counterarguments. This leads to more thorough case preparation, identification of novel legal strategies, and potentially better outcomes for clients.
Getting Started: Start with a small team of lawyers using Agentic RAG for research on complex cases, gradually expanding to larger teams as efficiency and accuracy improve.
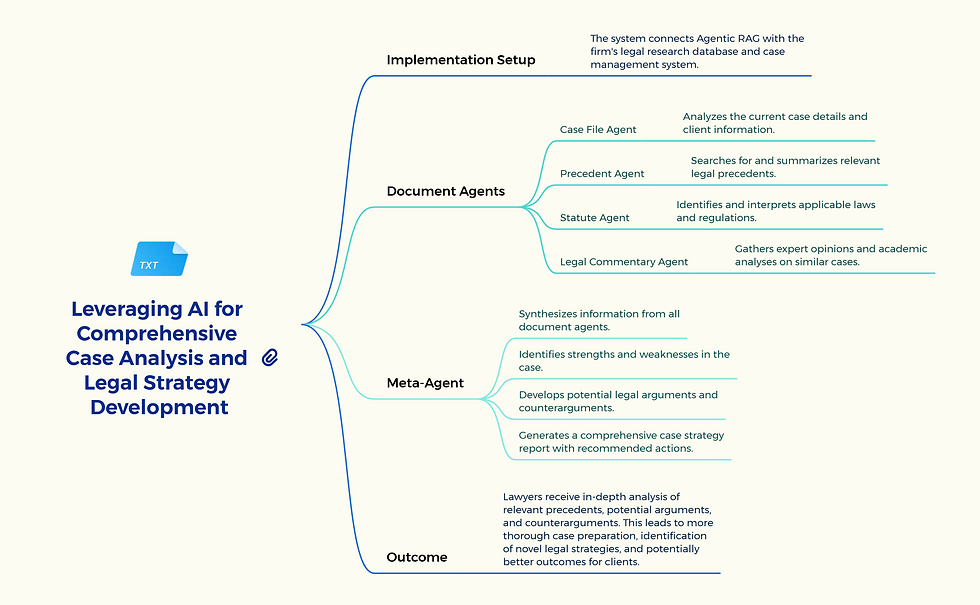
6. Financial Services Industry: Comprehensive market analysis and investment strategy formulation
Implementation Setup: Integrate Agentic RAG with financial databases and real-time market data feeds.
Document Agents:
Company Report Agent: Analyzes financial statements and annual reports of relevant companies.
Market Trends Agent: Tracks and interprets market indices and sector-specific trends.
Economic Indicator Agent: Monitors and analyzes key economic data.
News Agent: Scans and summarizes relevant financial news and analyst reports.
Meta-Agent:
Integrates data from all document agents.
Identifies correlations between economic indicators, market trends, and company performance.
Generates investment recommendations based on risk tolerance and market conditions.
Produces a comprehensive market analysis report with actionable investment strategies.
Outcome: Investment managers receive detailed market insights, potential investment opportunities, and risk assessments. This leads to more informed investment decisions, better risk management, and potentially higher returns for clients.
Getting Started: Use Agentic RAG for a trial run on a specific sector or portfolio, analyzing its impact on decision-making and returns.

7. Public Sector: Real-time emergency response coordination and resource allocation
Implementation Setup: Integrate Agentic RAG with emergency management systems, including data from first responders, hospitals, and weather services.
Document Agents:
Incident Report Agent: Analyzes real-time reports from first responders and emergency services.
Resource Availability Agent: Tracks the availability of emergency resources such as ambulances, fire trucks, and medical supplies.
Weather Data Agent: Monitors and interprets real-time weather data and forecasts.
Historical Incident Data Agent: Analyzes data from past emergency incidents to identify patterns and best practices.
Regulatory Guidance Agent: Summarizes relevant laws, regulations, and protocols for emergency response.
Meta-Agent:
Synthesizes information from all document agents.
Coordinates the allocation of resources based on real-time needs and availability.
Generates a comprehensive action plan with recommended response strategies and resource deployment.
Provides ongoing updates and adjustments based on new data and evolving situations.
Outcome: Emergency managers receive a detailed, real-time overview of the situation, enabling them to allocate resources efficiently and respond effectively to emergencies. This leads to faster response times, better resource management, and improved outcomes for those affected by emergencies.
Getting Started: Implement Agentic RAG in a specific emergency management department, such as fire and rescue services, to test its impact on response coordination and resource allocation.
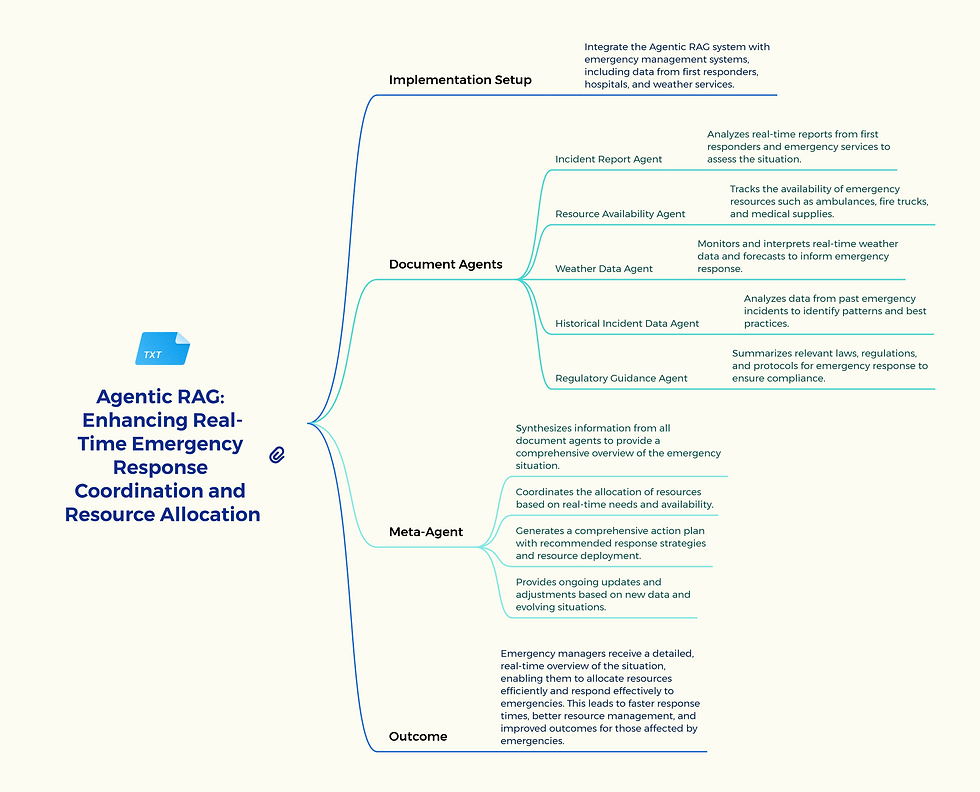
Conclusion
These examples demonstrate how Agentic RAG can significantly enhance decision-making, operational efficiency, user experiences, and public service capabilities. By leveraging the power of autonomous, adaptable, and proactive AI agents, organizations can unlock new levels of value and innovation. Start exploring how Agentic RAG can transform your business today!
——
Thank you for taking the time to read my thoughts. If my team can be of help, reach out to us at admin@drlisa.ai
Comments